How AI apps make money
📊 The state of AI pricing at 40 leading startups including ElevenLabs, EvenUp and Jasper
👋 Hi, it’s Kyle Poyar and welcome to Growth Unhinged, my weekly newsletter exploring the hidden playbooks behind the fastest-growing startups.
My most popular post this year is a deep dive into the state of SaaS pricing. As SaaS increasingly morphs into Al-infused applications, what’s the state of AI pricing?
For help I teamed up with Palle Broe, a startup operator and pricing & packaging expert for high growth tech companies. Palle is Director of Monetization Strategy & Growth at Series D-funded Templafy, early at Uber and author of the Rule of Thumb newsletter where he shares tangible advice for operators.
In the past few years there has been an explosion of AI products and applications—in fact, AI products already attract more than one-fifth of all VC funding.
Many of these AI apps change our relationship with software from something that makes us more productive to something that generates entirely new work products. This makes for favorable market conditions to create new innovative pricing strategies, which leaves us wondering: how are AI apps making money?
In the Large Language Model (LLM) space and the infrastructure layer, usage-based pricing models have been the norm since commercialization. Most of the companies charge on a per token basis, which is closely aligned with the cost of compute. We instead investigated 40 leading AI-native companies in the application layer to see what’s happening on the pricing front.
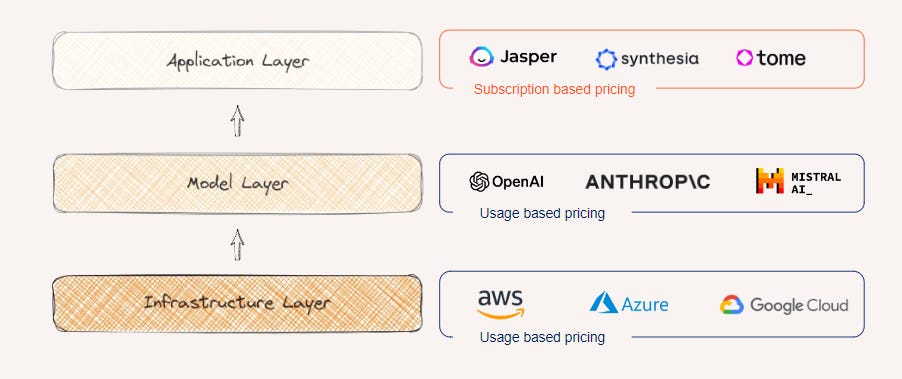
The primary criteria used to select the companies was based on external funding and traction from public sources like the Forbes AI 50 List and Sequoia’s Generative AI market map. The 40 companies include marketing tools (ex: Jasper, Copy.ai), productivity apps (ex: Tome, Glean), vertical specific products (ex: Harvey, Co:Helm), and others (ex: Synthesia, HeyGen).
Specifically, we reviewed public data focused around pricing models, value metrics, packaging, free versions and pricing transparency. We deliberately focused on AI-native apps rather than incumbents since these startups don’t have the baggage of a prior install base or legacy pricing model.
Pricing trends for 40 leading AI apps
Five findings stood out to us:
Limited pricing innovation—seven-in-ten have a subscription model and very few offer pure usage-based or pay-as-you-go pricing.
Most companies are charging based on the number of users—consistent with the notion of AI apps as “copilots” (assist people) rather than digital “workers”.
Free versions are extremely popular for initial adoption—one-in-two have a free plan, another one-in-five offer a free trial.
There’s a “Good-Better-Best” paradigm in terms of packages/tiers.
Varying degrees of pricing transparency—two-in-three have public pricing.
You can explore the full pricing data for yourself 👇
Lack of pricing innovation presents an opportunity for the second wave of AI apps
Software companies have historically leaned towards subscription and per-user model (even though there are signs of a shift to usage-based and hybrid models). This is still the case in the first wave of breakout AI apps.
We are seeing signs of very innovative pricing structures among the second wave of AI companies; these pricing models could unlock faster customer adoption while capturing more overall revenue. Heck, even Microsoft is testing innovative pay-as-you-go pricing for their new AI Copilot for Security.
Fin (Intercom), EvenUp, Chargeflow (an OpenView portfolio company), and 11x.ai — covered previously in Growth Unhinged — are examples of companies that have implemented success-based (or outcome-based) pricing models whereby a customer only pays for a successful outcome. For Chargeflow that’s a successful settlement, for Fin that is a successful resolution, and for EvenUp that’s a demand package successfully produced for a law firm (it’s worth listening to Sarah Tavel unpack how that works).
As a customer this is appealing as you are entering into win-win partnerships. The vendor is only successful if you are. This pricing also has the potential to increase adoption since customers only pay when the product delivers real results.
This is in stark contrast to a lot of the existing SaaS providers where customers often end up buying far more seats than they need. It is not uncommon to see monthly active user rates between 20-40%—where by definition customers are substantially over-paying for what they get. We expect the traditional per-seat subscription model will increasingly come under pressure as AI products deliver work rather than augment personal productivity.
Finding #1: Limited pricing innovation
Among the AI apps that we studied, the vast majority (71%) have adopted a traditional SaaS subscription pricing model. 10 companies (26%) have a hybrid pricing model combining both a subscription cost and a usage fee. PolyAI is the only company (3%) to have adopted a purely usage-based model.
While the infrastructure powering these applications are almost purely priced based on usage (LLMs and Infrastructure), this pricing has not translated to the end user products. We think there are a few reasons why:
Keeps it simple: The core objective for most AI applications is to get users to adopt their product. Buyers are familiar with paying a monthly fee per user.
Usage based pricing is hard to do: Let's be real—usage-based pricing is not easy to implement. It requires a strong data foundation, for instance, which is something many startups do not have early on.
Hard to quantify value yet: It's very likely that some of the AI apps are delivering value that way surpasses the price they are charging. But for many of the AI companies, it is still hard for them to understand exactly how much value they are creating and how they best capture it.
Don't want to limit adoption: Innovating on pricing can limit adoption of the product. Right now startups want to get as much usage as possible as they build out their products.
Not focused on profitability: Although the ZIRP days are over, the objective for a lot of the AI startups is not to be profitable, but to show that they can make money and grow with customers over time.
We do see some companies – particularly those within the marketing, video, and voice generation space – apply a usage based pricing component such as # of words, minutes of video characters or credits. Copy.ai makes for a good example.
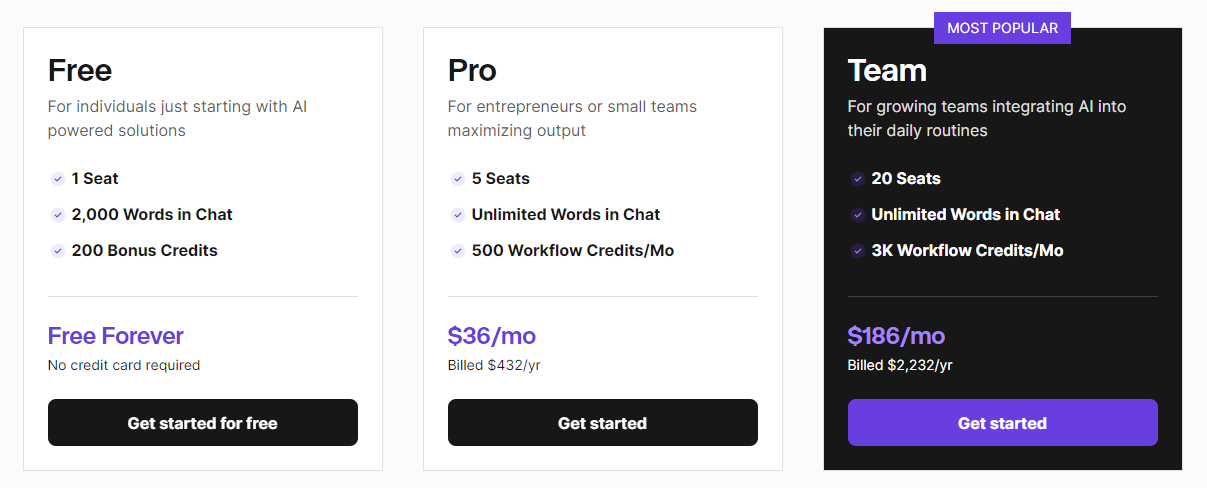
Finding #2: Most companies are charging based on the number of users
The primary value metric for the AI apps is still focused around users (or seats, if you will). This is a value metric that’s very well known in the SaaS world and is one of the most straightforward ways to buy and sell software with a high degree of predictability for buyers.
A dozen or so companies are using either both a per user and usage-based component or a pure usage-based model with value metrics like: credits, characters, minutes of video, subtitles or run-time.
Due to the nature of AI eventually replacing human labor, a per-user pricing model might be counter-effective as the number of users will get reduced over time. This presents a disruptive opportunity for the second wave of AI apps.
Finding #3: Free versions are popular for initial adoption
A variation of freemium is present in about 70% of the AI apps we studied. The three variations of freemium we saw were:
A free version (47%): A version that is “forever free” which often has limited capability but allows the user to try the feature/product.
A free version but limited in usage (3%): A version which is free but limited in the amount of usage of the product.
A free time-bound trial (16%): A version which is free and have basic capability but only for 7 or 14 days.
AI apps tend to have fast time-to-value for new users. Freemium offerings help generate early adoption and usage as these companies continue to iterate on the product.
Freemium is less prevalent among enterprise-focused applications. These products often involve implementation as well as platform fees in order to use them. Anecdotally, the enterprise equivalent of freemium appears to be free pilots where customers can try the product for a period of time (often 3 months) and then need to make a purchase decision.
Finding #4: There’s a “Good-Better-Best” paradigm in terms of packages/tiers
When we talk with early-stage startups, we normally recommend starting out with a variation of a “Good-Better-Best” packaging structure. It allows the company to differentiate offerings depending on the customer and creates a clear upsell path. The number of tiers varies by company from two tiers to five tiers (including freemium and an enterprise option). In most cases the differentiation between the tiers are based on product capabilities and usage.
Packaging strategies are often something that develops over time as the product matures and develops more functionality. Early on, you often don't have a lot to package as you don't know exactly who your customers are and how you should segment your product.
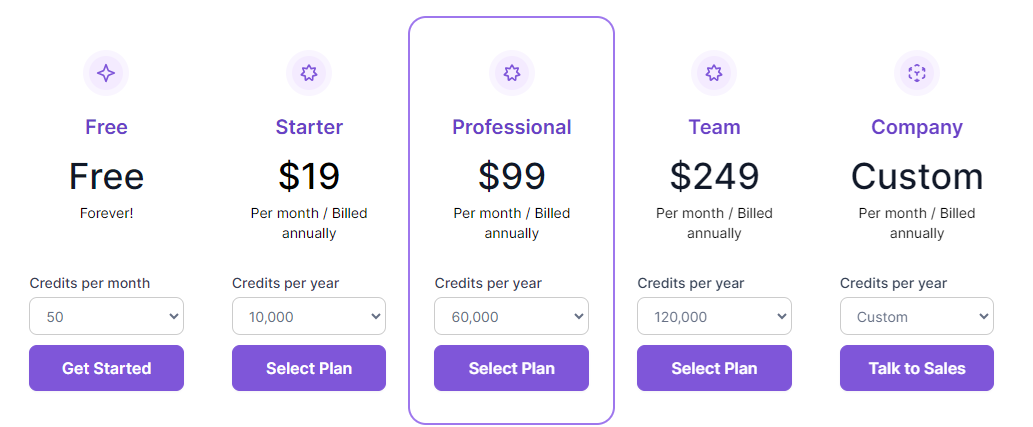
Finding #5: Varying degrees of pricing transparency
Around two-in-three companies are currently showing pricing on their website. Transparent pricing tends to be the norm for individual- or prosumer-focused apps rather than enterprise-focused ones.
Most enterprise AI apps did not share any details around their pricing. They are likely doing that for a few reasons:
It's a competitive space: Competition is heating up and companies don't want to give anything away to potential competitors or allow them to compete based on price.
Customization: They are customizing pricing from customer to customer and seeing where the upper bar is for what they can charge.
More flexibility: Many of these categories are still in their infancy and pricing isn’t yet locked in. Keeping pricing hidden offers more flexibility for vendors to change in the future.
In time, this pricing information will likely become public anyway given the rise of price benchmarking vendors such as Vendr or Tropic.
Final words
We’re in the very early innings of AI apps. Many are still seeking product-market fit (even if they’ve raised substantial capital) and looking to prove market demand. Innovating on pricing models is hard and is understandably not the core focus initially.
The name of the game right now seems to be: (1) make pricing predictable and (2) don't make it a barrier to using your product. Here’s a framework for figuring out where to start.
Thank You 🙏 Kyle
Very timely and well researched 🙌
Great article. It would be very beneficial if you could add to the main table the focus market for every AI app - BTB or BTC